Google has built the world's fastest ML training supercomputer and was able to set new records in six out of eight MLPerf benchmarks.
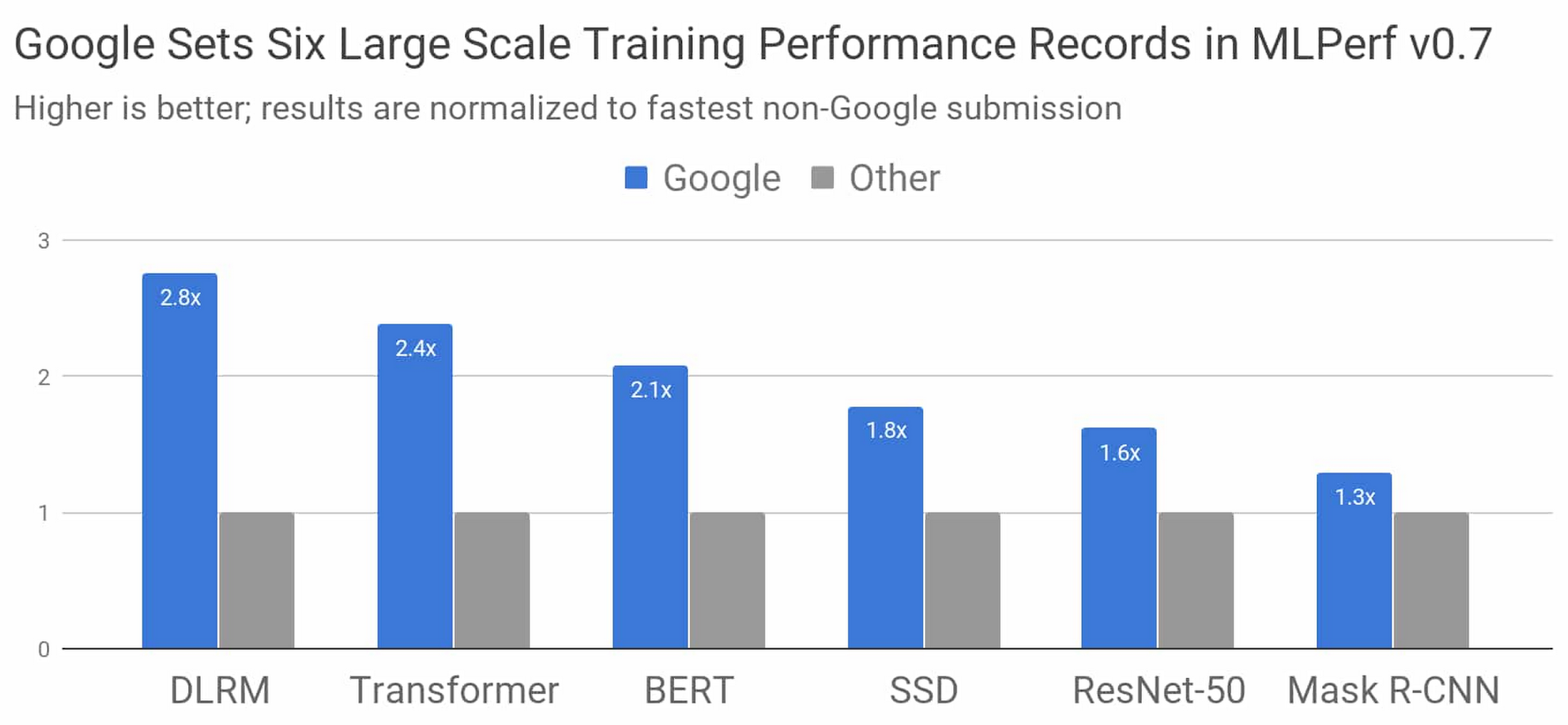
For example, they trained ResNet50 in 0.47 seconds. What does this really mean? According to Import AI newsletter:
Multi-year progress: These results show the time it takes Google to train a ResNet50 network to convergence against ImageNet, giving us performance for a widely used, fairly standard AI task:
- 0.47 seconds: July 2020, MLPerf 0.7.
- 1.28 minutes: June 2019, MLPerf 0.6.
- 7.1 minutes: May 2018, MLPerf 0.5.
- Hours - it used to take hours to train this stuff, back in 2017, even at the frontier. Things have sped up a lot.
You can read more about it from Google's Blog.
But What Does This Really Mean?
In a recent video posted by Lex Fridman, he talked about how the very hot GPT-3 compares to a human brain. Specifically, he told us that GPT-3 has 175 billion parameters and costs a whopping $4.6 million to train.So here's what it means:
Only tech giants like FAANG, Microsoft, and alike can afford the hardware and money to train large networks like this, and small startups/players really don't stand a chance.
You can learn what GPT-3 is in 3 minutes in this article.
So anyway, while we celebrate the advancement of AI, we also have to be careful about the increased impact capital has on innovation. I really do not want this to turn into something like our legal system where big corporations always win even though they "lose" the case or have to settle.
A pandemic does not just go away no matter how much you ignore it, deny it, or wish it would just magically disappear.
BTW: The easiest way to remember my blog address is http://blog.lannyland.com
nice tao of the day
ReplyDelete